The Rise of Custom AI Chips: How Big Tech is Challenging NVIDIA’s Dominance
Published on 28 Apr, 2025
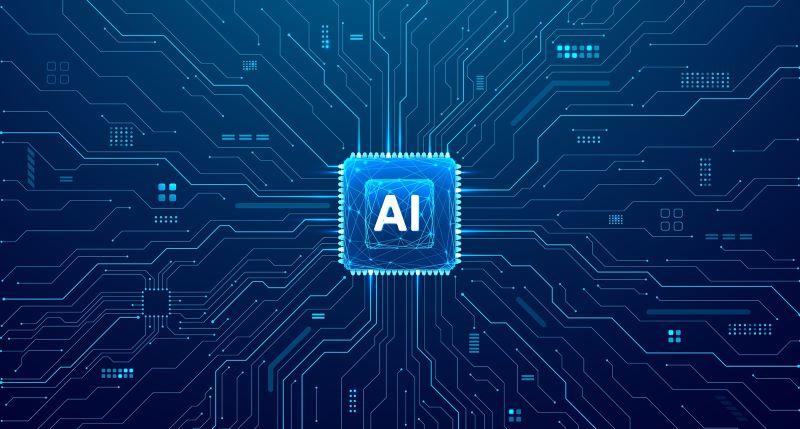
Big Tech is ramping up efforts to reduce dependence on NVIDIA by designing custom AI chips tailored to their workloads. Google, Amazon, Microsoft, and Meta are deploying these chips across their data centers to optimize cost, performance, and energy efficiency. This move supports tighter integration with their cloud ecosystems, enabling greater control over AI infrastructure and enhancing long-term scalability. While NVIDIA remains the market leader, these developments mark a strategic shift toward vertical integration. Our latest analysis explores how in-house AI chips are reshaping the competitive dynamics in the semiconductor and AI markets—and what this means for the technology sector.
The AI chip market—expected to reach $91.96 billion by 2025—has long been dominated by NVIDIA’s general-purpose GPUs, notably the H100. However, tech giants such as Google, Amazon, Meta, and Microsoft are increasingly investing in custom silicon designed to meet the performance, efficiency, and scalability demands of their cloud platforms and internal AI workloads. This article explores the development of proprietary chips such as the TPU, Trainium, Inferentia, MTIA, and Maia, examining how they compare with NVIDIA's flagship GPU, and identifying key trade-offs, strategic motives, and market implications. We argue that these custom solutions are fragmenting the AI hardware space, promoting a shift toward ecosystem-optimized, cost-effective, and use-case-specific architectures.
1. Introduction
The rise of artificial intelligence (AI) has created an insatiable demand for high-performance computing hardware. For much of the last decade, NVIDIA has led the AI chip market, with its H100 GPU setting benchmarks in performance, scalability, and software support through its CUDA platform. However, a new trend has emerged: vertical integration via custom AI chips developed by major cloud service providers and platform companies.
Google (TPU), Amazon (Trainium, Inferentia), Meta (MTIA), and Microsoft (Maia) are building domain-specific architectures (DSAs) to reduce costs, improve efficiency, and gain independence from third-party suppliers. These chips reflect a strategic shift—from general-purpose computing to application-specific acceleration, tailored for hyperscale environments and proprietary AI stacks.
2. Development and Technical Overview
The development of custom AI chips by big tech firms diverges sharply from NVIDIA’s GPU-centric model. While NVIDIA prioritizes flexibility and raw compute power, these companies focus on efficiency, integration, and targeted performance.
Company | Chip | Key Specs | Focus |
---|---|---|---|
TPU v5p | 459 TFLOPS (bfloat16), systolic array architecture | Matrix-heavy AI tasks, energy efficiency | |
Amazon | Trainium & Inferentia | Up to 2,000 TOPS (INT8), TSMC 7nm/5nm | Cost-efficient training and inference |
Meta | MTIA v2 | 3x performance over v1, optimized for sparse models | Internal recommendation systems |
Microsoft | Maia 100 | ~105 billion transistors (details sparse) | Azure AI scalability |
NVIDIA | H100 | 4 PFLOPS (FP8), 141 GB HBM3, 700W TDP | Mixed-precision general AI workloads |
Insight: Big tech chips often trade peak FLOPS for power efficiency and task-specific optimization. DSAs (e.g., TPUs, MTIA) are leaner and more efficient than general-purpose GPUs but offer limited flexibility—an acceptable compromise for hyperscalers with predictable workloads.
3. Marketability Strategies
Each company’s approach reflects its broader business model:
- Google: TPUs are exclusive to Google Cloud, with tight integration into TensorFlow. Marketing emphasizes performance-per-watt and developer ease.
- Amazon: AWS uses Trainium and Inferentia to disrupt pricing, claiming up to 50% cost savings over GPUs, making them attractive to inference-heavy customers.
- Meta: MTIA is currently internal, focused on Meta’s ad infrastructure. Future commercialization could target social media and e-commerce players.
- Microsoft: Maia chips are positioned as turnkey solutions for Azure clients, enhancing enterprise appeal and supporting the company's OpenAI integration.
- NVIDIA: The H100 is sold across sectors—from research to hyperscale—backed by CUDA, a universal, mature programming ecosystem.
Insight: A key divide lies in business models: big tech offers AI compute as a service (rental), while NVIDIA follows a hardware ownership model. The former drives ecosystem lock-in and recurring revenue, while the latter provides more flexibility for advanced users.
4. Customer Appeal: Why Choose Big Tech Over NVIDIA
While NVIDIA leads in peak compute, many customers prioritize:
- Cost Efficiency: Amazon claims up to 50% savings using Inferentia vs. GPUs. TPUs offer similar benefits by reducing energy consumption and operational costs.
- Ecosystem Integration: Seamless alignment with existing cloud platforms (e.g., Azure, Google Cloud) reduces onboarding friction and enhances manageability.
- Workload-Specific Performance: MTIA excels in sparse, recommendation-heavy workloads, where H100’s raw power may be underutilized.
- Cloud Convenience: Renting compute removes the need for capex and in-house hardware maintenance, especially appealing to startups.
- Sustainability: Lower TDPs (e.g., 200W for TPUs vs. 700W for H100) support ESG goals and reduce data center overhead.
Insight: Smaller firms prioritize simplicity and cost; larger enterprises with custom needs may still prefer NVIDIA for programmability and control. That said, cloud-native AI startups are increasingly favoring proprietary cloud silicon.
5. Comparative Analysis
Factor | Google TPU v5p | AWS Trainium/Inferentia | Meta MTIA | Microsoft Maia | NVIDIA H100 |
---|---|---|---|---|---|
Performance | 459 TFLOPS (bf16) | Up to 2,000 TOPS (INT8) | 3x previous gen | TBD (105B transistors) | 4 PFLOPS (FP8) |
Power Efficiency | High (~200W) | Moderate-High (~150W) | High (sparse-optimized) | TBD | Moderate (~700W) |
Cost Advantage | High | Very High | Internal only | Moderate | Low |
Ecosystem Fit | Google Cloud | AWS | Meta internal | Azure | Broad (on-prem & cloud) |
Programming Stack | TensorFlow | AWS SDK | Custom tools | Azure ML/td> | CUDA |
Insight: Custom silicon outperforms GPUs only in well-defined scenarios. Power efficiency and integration win out over peak compute in inference and large-scale deployment environments.
6. Discussion
Big tech’s foray into custom AI chips is part of a broader strategic play for vertical integration and cost control. Estimates suggest:
- Google’s TPUs reduce internal cloud costs by 20–30%.
- Amazon’s silicon helps AWS protect its 32% market share in cloud.
- Meta invests over $10B annually in AI, with MTIA improving inference throughput.
- Microsoft uses Maia to strengthen its position via OpenAI and Azure ML.
These chips are not intended to universally replace NVIDIA, but to dominate within tightly integrated ecosystems, gradually fragmenting the market.
New Insights:
- Technical Trade-Offs: Focus on INT8/bfloat16 improves throughput and power use but limits use in precision-sensitive domains (e.g., scientific research).
- Market Trends: Edge AI and sustainability favor custom DSAs. NVIDIA’s Jetson series addresses edge, but still lags in energy efficiency.
- Lock-In Risk: Using big tech chips ties firms to proprietary stacks, risking innovation stifling compared to open hardware/software ecosystems.
- Challenges: Big tech lacks NVIDIA’s decades of hardware design expertise. Software support and community ecosystems remain less mature.
7. Conclusion
Big tech companies are carving out a new path in AI hardware, favoring customization over generality, and efficiency over brute force. While NVIDIA’s H100 remains dominant for now, Google, Amazon, Meta, and Microsoft offer compelling alternatives within their ecosystems. These chips are:
- Affordable (e.g., Trainium),
- Tightly integrated (e.g., Maia with Azure),
- Optimized for specific workloads (e.g., MTIA for recommendations),
- And energy efficient (e.g., TPU v5p).
This suggests an emerging equilibrium: NVIDIA will lead in general-purpose AI compute, while big tech will excel in specialized cloud-native AI delivery. As the market grows and diversifies, future developments in edge AI, generative models, and sustainable computing will further define this evolving competitive landscape.