Gearing up for the Next Wave of AI in Pharmaceutical Industry – From Cost-based to Value-based models
Published on 11 Jun, 2019
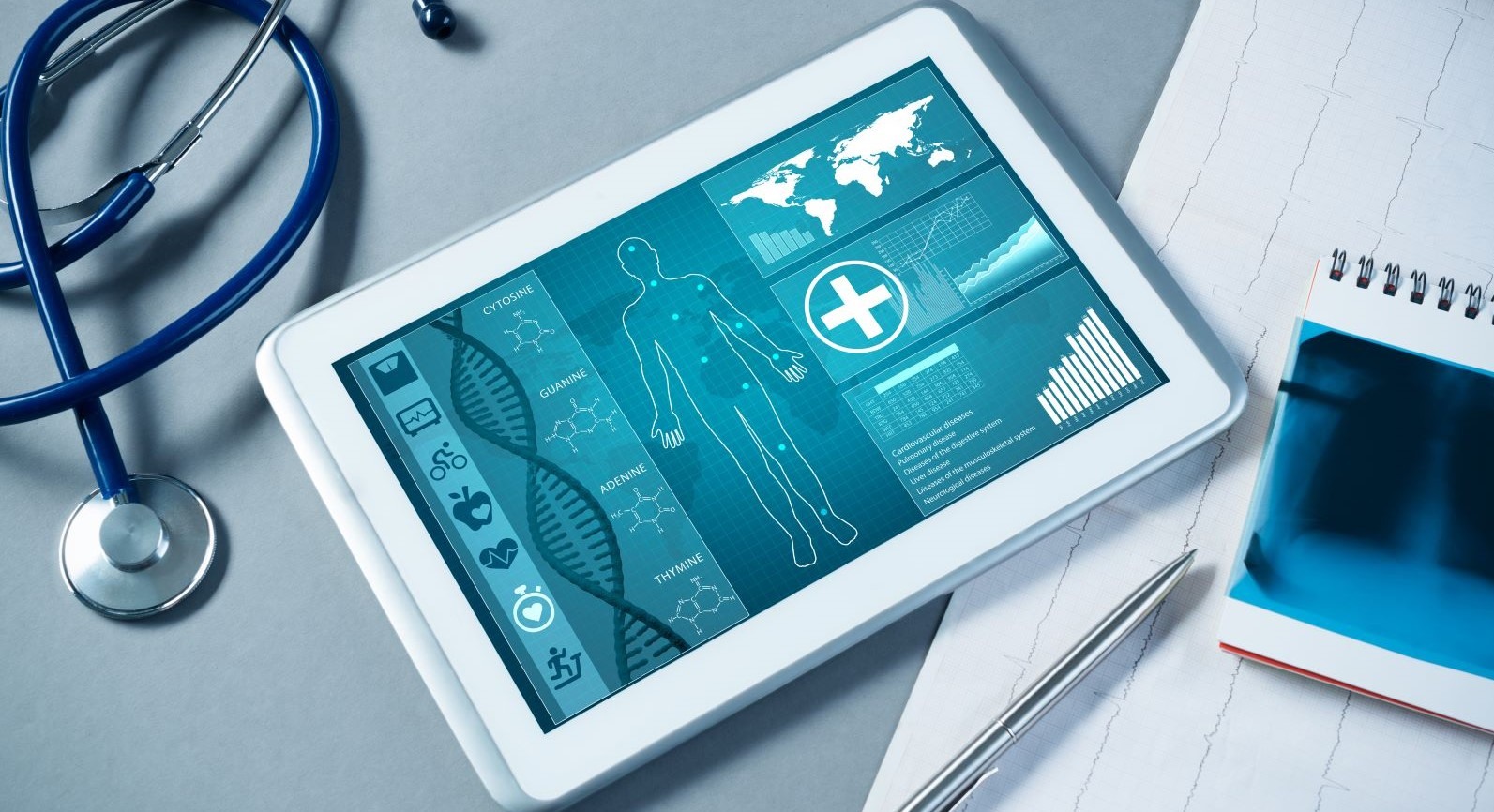
Artificial intelligence (AI) is reshaping business operations in the health industry. Amid the ever-evolving AI infrastructure, growth in tech-savvy patients and availability of big data, the industry is set to make a transition from using AI for controlling costs to utilizing it for improving patient care. The AI in healthcare market is estimated to reach $6.6Bn in 2021 from $600Mn in 2014, recording a CAGR of 40%; in the five years subsequent to 2021, the market is estimated to grow by more than 10 times. In this article, we provide insights on the applications of AI in patient-centric models for improving patient care, from diagnosis to post-treatment care.
Over the last few years, initial applications of AI in the pharmaceutical business have been in the areas of drug discovery – predicting molecule-target bonding, identifying biomarkers, uncovering new drug indications, conducting clinical trials and improving manufacturing efficiencies. While the cost of developing a drug could reach ~$2.5 Bn and it could take 10–15 years to complete phase 3 clinical trial, the use of AI, it is estimated, is likely to reduce the cost by around 70%. AI is increasingly gaining traction in other areas, including commercial operations, and collection, synthesis and use of data.
Next Wave
Given the efficiencies associated with AI, it is likely to be adopted at a large scale in the highly regulated pharmaceutical industry. It is expected to help the pharma sector solve the complex issue of adding value and improving the patient healthcare outcomes, cost effectively, through the upcoming years of patent cliffs and increasing generic competition. According to estimates, companies will spend $54Mn on an average on AI projects by 2020, which could generate savings of over $150Bn for the healthcare industry by 2025.
Intensive use of big data in healthcare, coupled with the increasing AI skillset, would boost the adoption of AI for newer applications. Entry of IT giants in healthcare, increased adoption of AI technologies by big pharma companies, and collaborations of pharma companies with tech and IT startups would also contribute to this. Around $2.7Bn was raised in 206 deals between 2011 and 2017 by 121 health AI and machine learning companies.
The next wave of AI in the pharmaceutical industry would be focused on patient-centric models for improving every aspect of patient care, from diagnosis to post-treatment. Learnings and insights drawn from advancements in the health tech and medical device industry are now being applied to real-time applications in the pharma industry to raise the quality of patient care.
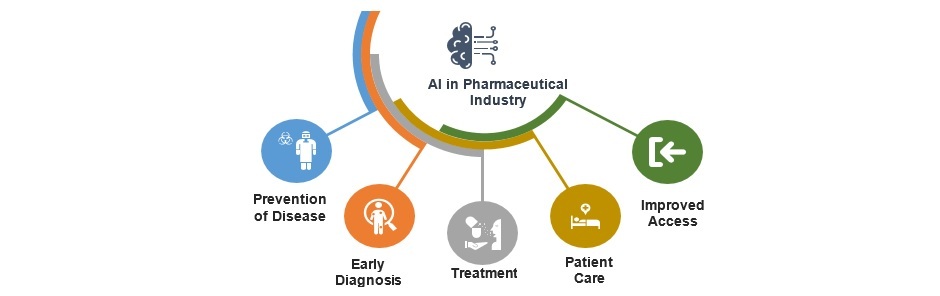
- Prevention
Predictive medicine is a growing trend in the healthcare domain. Using data from medical sensors and genomics, medical professionals are now able to draw conclusions about the risk factors of diseases.
A case in point is Scripps Research Translational Institute and Nvidia, an AI company, collaborating to develop AI and deep learning solutions for deriving insights from genomics and health sensor data. The partnership will initially focus on developing deep learning tools to predict atrial fibrillation as well as analytics of whole genome sequences. Thereafter, the scope will be expanded to include other diseases and datasets.
Machine learning can help in assessing the risk of getting a heart disease. Google and Verily have developed an algorithm, which analyzes the scans of the back of the eye to deduce a person’s age, his blood pressure, and smoking habit and, accordingly, predict the risk of a major cardiac event.
-
Early Diagnosis
Data on Electronic Health Records (EHR), smartphones, smart watches and other tracking devices has been used to evaluate patients in real time and determine their health status. For example, the FDA has cleared Apple Watch ECG, which is helpful in diagnosing heartbeat abnormalities. The electrocardiogram app is available without a prescription for people aged 22 and above. Apple obtained a second FDA clearance for an app that identifies irregular heart rhythms that may indicate atrial fibrillation.
The table below highlights a few more FDA approvals for AI platforms majorly used for early diagnosis:
Company Approval Indication Apple
September 2018
Atrial fibrillation detection
Aidoc
August 2018
CT brain bleed diagnosis
iCAD
August 2018
Breast density via mammography
Zebra Medical
July 2018
Coronary Calcium scoring
Bay Labs
June 2018
Echocardiogram EF determination
Neural Analytics
May 2018
Device for paramedic stroke diagnosis
IDx
April 2018
Diabetic Retinopathy Diagnosis
Icometrix
April 2018
MRI brain interpretation
Imagen
March 2018
X-ray wrist fracture diagnosis
Viz.ai
February 2018
CT stroke diagnosis
Arterys
February 2018
Liver and Lung Cancer (MRI,CT) diagnosis
MaxQ-AI
January 2018
CT brain bleed diagnosis
Alivecor
November 2017
Atrial fibrillation detection via Apple Watch
Arterys
January 2017
MRI heart interpretation
Timely deployment of AI could prove beneficial in early diagnosis and treatment. Deep learning models developed to study metabolic changes in the brain could help in early detection of Alzheimer’s (at least six years before clinical diagnosis of the disease) and pave the way for prompt treatment. Also, work on a deep learning model, capable of diagnosing tumors at a success rate of 92%, is underway.
-
Treatment
Robotics is widely used in healthcare, from doing surgeries to supporting self-management of patients with long-term conditions. AI-assisted robotic surgeries could result in five-fold reduction in surgical complications and a 21% decrease in patients’ length of stay in the hospital following a surgery.
In the pharmaceutical industry, AI is being used to improve patient outcomes by evaluating patient data and prioritizing treatment urgency for patients in hospital settings. AI-empowered frameworks are being used to understand outcomes following treatment and recognize ideal medications depending on patients' profiles. Thus, efficient clinical decision-making will facilitate treatment customization.
-
Patient Care
Application of AI in improving patient care will enable healthcare companies to understand patient requirements closely and design products accordingly. By collecting and analyzing aggregate anonymized patient level data (APLD) across a patient’s journey, pharma companies can gain deeper insights on the behavior, journey and unmet needs of patients.
A case in point is improvement in drug compliance. Real-time patient data can be used to generate reminder for a missed dose.
The National Institutes of Health (NIH) has developed the AiCure app, which helps patients in monitoring their medications. The webcam of a smartphone is integrated with AI to manage medicines for the patient. The application generates self-governing confirmation on whether a patient is regularly consuming the prescribed medications or not. Medication compliance is essential for the success of every treatment and AI can unquestionably provide a benefit there. A 28-patient study in the American Heart Association's journal Stroke shows that AiCure's AI platform led to a 50% improvement in adherence to oral anticoagulants.
-
Access
Use of real world evidence (RWE) and patient-level data for confirming the clinical outcomes of drugs will aid in policy decision making and thus may improve access to essential drugs. For example, Novartis has used retrospective real world evidence studies to reconfirm the clinical outcomes of its drugs such as Cosentyx (Secukinumab) and Revolade (Eltrombopag) which were initially proved through clinical studies. This has helped the company gain a better understanding on the outcomes of treatments and, thus, help healthcare providers navigate treatment options more effectively.
Health economics and outcomes research (HEOR) analysts can extensively use machine learning algorithms on patient data to arrive at better decisions on reimbursement needs and drug pricing which could help in greater patient access.
With newer applications of AI on the horizon, the pharmaceutical industry could make a paradigm shift toward customized and cost-effective patient value models. On the flip side, the industry needs to watch out for the considerable threats AI could pose: privacy issues, ethics-related concerns, medical errors, and loss of human touch in patient care. Pharmaceutical industry will need to ensure quick adoption of upcoming applications and bring a change in the cost-based mindset. Industry skills need to be upgraded to overcome challenges such as those related to unstructured or inconsistent data and measurement of returns on investment in AI. AI has its pros and cons, depending on the perspective: while it can significantly enhance the quality of healthcare and alter the domain, it could result in severe job loss. Balancing the risks and rewards of AI in the pharmaceutical space will require collaborative effort from technology developers, regulators, medical fraternity, pharmaceutical companies and patients.